Propensity modeling has emerged as a powerful tool in data analytics and customer behavior prediction. It’s a statistical approach that helps businesses predict the likelihood of specific customer actions.
But what exactly is propensity modeling, and how can it be applied in real-world scenarios? Let’s dive in.
What is Propensity Modeling
In simple terms, propensity modeling is like a crystal ball for businesses. It’s a tool that uses past data and statistics to predict how likely a customer will do something in the future. This “something” could be buying a product, clicking on an email, or even stopping service use. Using propensity modeling, businesses can make better decisions and strategies to encourage positive customer actions and prevent negative ones.
What are the different types of propensity models?
There are several types of propensity models, each predicting a different kind of customer behavior. Here are a few examples:
- Propensity to Buy or Convert: This model predicts how likely a customer is to make a purchase or take a desired action (like signing up for a newsletter). It’s like a fortune teller for sales, helping businesses know who might be ready to buy and who might need a little more convincing.
- Propensity to Unsubscribe: This model is like a crystal ball for email marketers. It predicts who is likely to unsubscribe from an email list. With this information, businesses can try different strategies to keep these people interested and engaged.
- Propensity to Churn: This model predicts which customers are likely to stop using a service or product. It’s like a weather forecast for customer loyalty, helping businesses identify who might be drifting away so they can take action to keep them.
Each of these models helps businesses anticipate customer actions and make smarter decisions.
Why Should Optimizers Care About Propensity Modeling?
Understanding the mathematics behind propensity modeling is crucial for optimizers. It’s akin to how SEO experts need to understand a bit of content marketing and HTML to be competent. Propensity modeling offers a way to predict customer behavior, which can inform strategies for testing and experimentation.
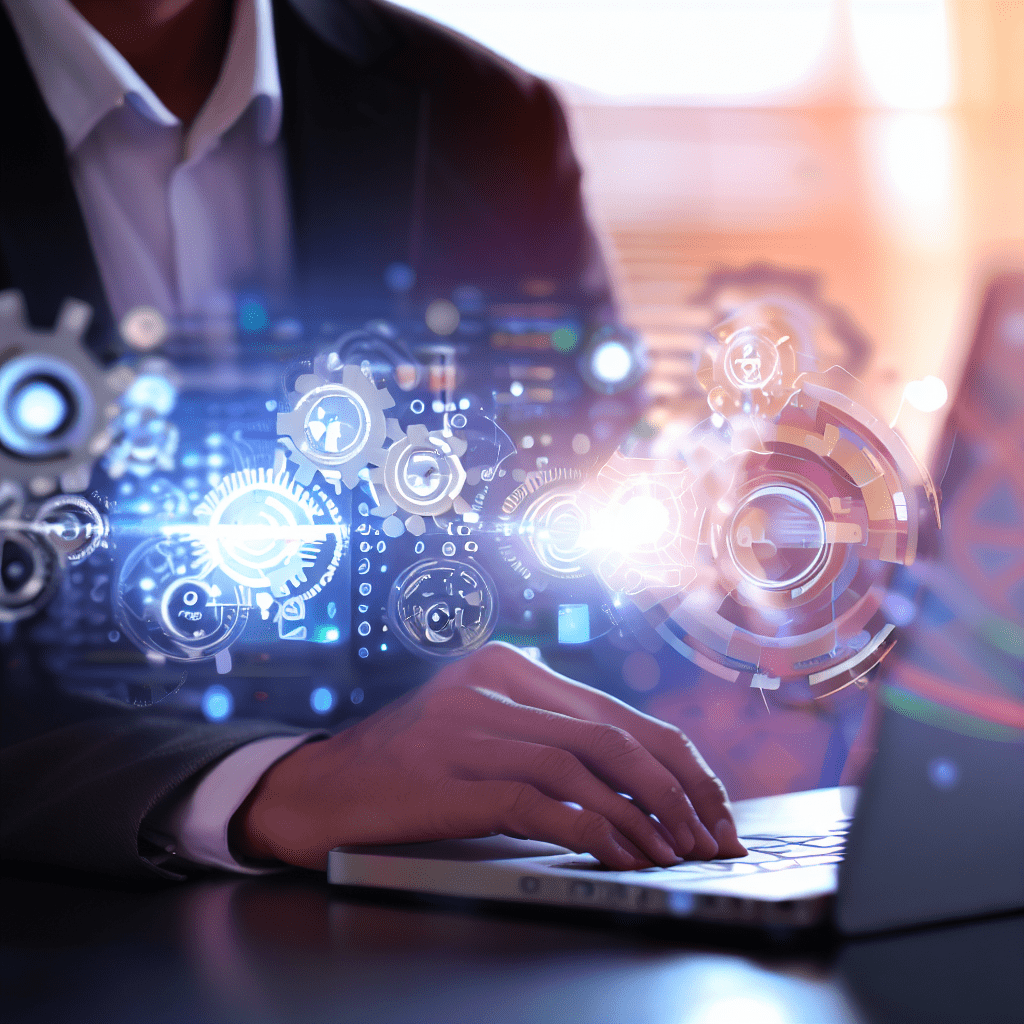
What is the difference between uplift model and the propensity model?
Both uplift and propensity models are used in predictive analytics, but they serve different purposes and answer different questions.
Propensity models are like fortune tellers for individual customer behavior. They predict how likely a customer is to take a certain action, like purchasing or unsubscribing from an email list. The question they answer is, “How likely is this customer to do X?”
On the other hand, uplift models are more like guides for the effects of a specific action or treatment on customer behavior. They predict the change in a customer’s behavior due to a particular action taken by the business, like sending a promotional email or offering a discount. The question they answer is, “How much will doing X influence this customer’s behavior?”
In other words, while propensity models predict the likelihood of an event, uplift models predict the treatment’s influence on that event’s likelihood.
How to do propensity modeling
Remember, propensity modeling is a complex process involving advanced statistics and data science skills. But with the right tools and expertise, it can be a powerful way to understand and predict customer behavior.
Here’s a simplified step-by-step guide on how to do propensity modeling:
- Identify Your Goal: The first step is to decide what customer action you want to predict. This could be anything from making a purchase to unsubscribing from an email list.
- Select Your Features: These are the variables that you think might influence the action you’re trying to predict. For example, if you’re predicting purchase behavior, features might include a customer’s browsing history, previous purchases, and demographic information.
- Collect and Prepare Your Data: Gather historical data for the features you’ve selected. This might involve pulling data from various sources and cleaning it to ensure it’s accurate and usable.
- Choose a Model: Depending on your goal and the nature of your data, you’ll choose a statistical model. This could be a type of regression, a decision tree, or another type of model.
- Train Your Model: Use your historical data to train your model. This involves running your data through the model and adjusting the model’s parameters until it can accurately predict the outcome based on the features.
- Test Your Model: Once your model is trained, test it on a different set of data to see how well it predicts the outcome. This helps ensure that your model will work well when you use it in the future.
- Use Your Model: Now that your model is trained and tested, you can use it to predict customer actions. The predictions are probabilities, so they’re not 100% certain. But they can give you a good idea of what to expect from your customers.
- Refine Your Model Over Time: As you collect more data and learn more about your customers, you can refine your model to make it more accurate.
Using Your Propensity Model for Smarter Experimentation
Propensity modeling and experimentation are not mutually exclusive. In fact, they work best when combined. For instance, propensity modeling can help fill your pipeline, save time on quantitative conversion research, and explore smarter segmentation options. However, it’s important to remember that correlation does not imply causation. Just because two variables are correlated in your model doesn’t mean one causes the other.
Propensity Modeling with Machine Learning Model
Let’s delve deeper into why an experienced data science team is recommended for enterprise-scale companies in propensity modeling.
Pre-trained machine learning solutions are often designed to be general-purpose tools. They are trained on a wide range of data and intended to work reasonably well across many scenarios. However, this general-purpose design can be a limitation. These models might not account for the nuances and unique factors relevant to a particular business or industry.
For instance, a pre-trained model might not consider certain industry-specific factors or unique customer behaviors crucial for predicting propensities in a specific business context. This could lead to less accurate predictions, which could impact the effectiveness of business strategies based on these predictions.
On the other hand, an experienced data science team can build custom propensity models that are specifically tailored to the needs of the business. They can select and engineer relevant features to the specific business context, train models on company-specific data, and continually refine these models based on new data and insights. This can lead to more accurate and reliable propensity scores.
Moreover, a dedicated data science team can also ensure that the models are scalable and robust, handling large volumes of data and providing reliable predictions even as the business grows and evolves. They can also implement proper model maintenance practices, regularly checking the performance of the models, updating them as needed, and ensuring they continue to provide value as business needs change.
In summary, while pre-trained machine learning solutions can provide a starting point for propensity modeling, enterprise-scale companies that require more accurate, robust, and scalable models would benefit from the expertise of a dedicated data science team.
The Role of Propensity Modeling in Predicting Customer Behavior
Propensity modeling is a powerful tool for predicting customer behavior. It can help businesses anticipate customer actions and tailor their strategies accordingly. For instance, if a propensity model predicts a high likelihood of a customer churning, the business can take proactive steps to retain that customer.
The Intersection of Propensity Modeling and Experimentation
While propensity modeling provides valuable insights, it’s not a replacement for experimentation. The two can complement each other. For instance, you can use propensity modeling to inform your experimentation strategies, and vice versa. The key is to use each tool where it adds the most value.
Propensity to Buy or Convert
Understanding the propensity to buy or convert can be extremely valuable for businesses. This model predicts how likely visitors, leads, and customers are to make a purchase or convert to the next step of the funnel. Those with a lower propensity score may need more incentives than others. For instance, an ecommerce store might offer a higher discount to those with a lower propensity to buy.
Propensity to Unsubscribe
The propensity to unsubscribe model predicts how likely recipients, leads, and customers are to unsubscribe from your email lists. To those with a higher propensity score, you might try reducing the frequency of emails or sending a special offer to reinforce the value of remaining a subscriber.
Propensity to Churn
Identifying at-risk leads and customers is another crucial application of propensity modeling. If they have a high propensity score, you might experiment with in-product win-back campaigns or assign account concierges to reconnect them with your core value proposition.
The Value of Propensity Modeling in Optimization
When combined with optimization expertise, propensity modeling can lead to smarter, more targeted experiments yield more valuable, transferable insights. It pushes your data beyond what has already happened and toward what is likely to happen in the future.
Conclusion
Harnessing the power of data, propensity modeling allows marketing teams to build a crystal ball for customer behavior. This modeling technique uses real-world data to predict whether a customer will convert, purchase a product, or even unsubscribe. By analyzing past behaviors with a supervised machine learning algorithm, we can calculate propensity scores that help identify the likelihood of different outcomes. Propensity models can analyze data, forecast behavior, and even predict binary outcomes like whether an undecided voter could swing.
So, dive into the world of predictive propensity modeling, use the data at your fingertips, and let your business thrive in the era of data-driven decisions.
Answering your questions about customer propensity model
Q: What is propensity modeling?
A: Propensity modeling is a technique used in data science and analytics to predict an individual’s likelihood of taking a particular action or behavior based on historical data. It involves building a predictive model to forecast the propensity of a person to perform an action or behavior.
Q: What are the different types of propensity models?
A: There are various propensity models, such as logistic regression models, decision trees, random forest models, and machine learning algorithms.
Q: What is the use case of propensity modeling?
A: Propensity modeling finds extensive use in marketing campaigns to identify potential customers who are more likely to purchase specific products or services. It helps marketers improve their campaigns’ efficiency by targeting those with a higher probability to convert.
Q: How does propensity modeling work with machine learning?
A: Propensity modeling with machine learning involves leveraging machine learning algorithms to create more accurate predictive models. Machine learning algorithms learn from the data and identify relevant features automatically, making the model more flexible and adaptable to new data.
Q: How can I implement propensity modeling?
A: You can implement propensity modeling by following a few basic steps, such as identifying the relevant data, training the model, and using this model to predict the propensity of new data. Establishing a relevant metric to evaluate the model output and ensure its effectiveness is also essential.
Q: Can I use propensity models for a real-world application?
A: Yes, propensity modeling is widely used in various industries, such as banking, insurance, retail, and healthcare, to help organizations make data-driven decisions and improve customer experiences.
Q: How do I use Google Analytics data for propensity modeling?
A: Google Analytics can provide valuable data that can be used to train a propensity model, such as user demographics, behavior, and engagement metrics. It’s essential to select the relevant data for the model and transform it into a format that the model can understand.
Q: Why is training data essential in propensity modeling?
A: Training data is crucial in propensity modeling as it helps the model learn patterns and relationships between features and target variables. It’s essential to have enough relevant training data to ensure the accuracy of the model.
Q: What is customer lifetime value (CLV) in propensity modeling?
A: Customer lifetime value (CLV) is a metric used in propensity modeling to calculate the total value a customer will bring to a business over a certain period. It’s a critical factor in identifying valuable customers and predicting their propensity to buy products or services.
Q: How is propensity modeling different from predictive modeling?
A: Propensity modeling is a form of predictive modeling, but a specific type of prediction. Propensity modeling focuses on predicting an individual’s likelihood to perform a specific behavior, while predictive modeling predicts future outcomes or events based on historical data.